Everyone's talking about how AI is transforming translation — but can it really handle the nuances of human language?
Recent research by Bureau Works put this to the test, comparing GPT-3 with major machine translation engines from Google, Amazon, and Microsoft. The study focused on one of the toughest challenges in localization: idiomatic expressions. Why? Because literal translations don’t cut it when you're dealing with phrases like “The cat’s out of the bag” or “Let’s call it a day.”
The results were surprising. GPT-3 delivered accurate, natural-sounding translations up to 90% of the time, while traditional engines hovered between 20% and 50% — and often produced cringeworthy outputs rated “I want to cry” by native linguists.
In a world where linguistic nuance can make or break your message, these findings highlight both the promise and the current limitations of AI in professional translation.
Here is a Summary of Our Key Findings:
- GPT-3 consistently outperformed traditional MT engines (Google, Amazon, Microsoft) in handling idiomatic expressions — reaching up to 90% accuracy in languages like Portuguese and Chinese.
- Traditional engines struggled with metaphor and figurative language, often delivering literal translations that were grammatically correct but semantically off — some even earning “I want to cry” ratings from native reviewers.
- Microsoft took more risks, offering bolder translations that sometimes deviated from the original but felt more natural — though GPT had a harder time evaluating these creative choices.
- GPT’s qualitative analysis was strong, but it struggled to assign consistent numerical scores, especially when differentiating between mid-range ratings like 3 vs. 4.
- In almost every language, GPT’s second iteration — when prompted for a more figurative version — improved clarity, tone, and cultural fit.
- Korean was the most challenging language, both for traditional MTs and GPT-3, showing significantly lower performance across the board.
- GPT-3 proved valuable not only as a translator but also as a tool for evaluation and revision, helping flag awkward or inaccurate phrasing and offering alternatives.
GPT Achieved 90% Accuracy in Chinese Idioms—MTs Struggled
The following analysis was written by our English/Chinese linguist James Hou.
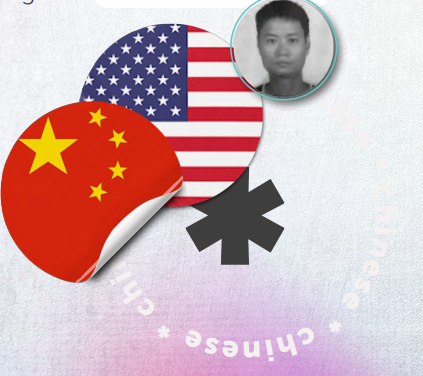
Overall all engines struggled with the metaphorical nature of language, often erring in excessive literality. For example, The translation “让我们称之为一天。” is a more literal and somewhat awkward translation of “Let’s call it a day.” It conveys the meaning of the original phrase, but the wording is somewhat awkward and may not be as easy for native Chinese speakers to understand.
While it accurately conveys the meaning of the original phrase, the wording is somewhat awkward and may be less clear to native speakers.
Output from a Qualitative Perspective
The table below presents a synthetic analysis of 10 English idiom sentences translated into Chinese, as conducted by our team of linguists.
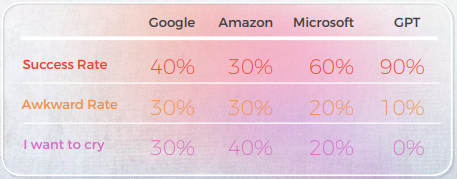
As far as translation quality goes, GPT did a great job with contextualization. For example, “秘密被揭露了。” is an accurate and clear translation for “The cat’s out of the bag”. In 9 out of 10 sentences, the content was well adapted, intelligible, and conveyed the appropriate meaning. Contrary to the three Machine Translation engines, GPT had no embarrassing “I want to cry” mistakes.
The table below contains the raw data analysis.

Evaluation of GPTs Evaluation
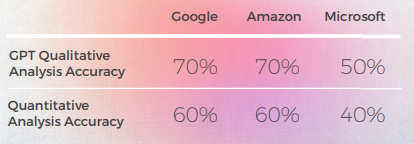
Analysis and Key Findings
- Google and Amazon had extremely similar results, only slightly deviating from each other, mirroring each other’s mistakes and metaphorical choices. For example, the translation “打我。” is not an accurate or appropriate translation of “Hit me up.” The phrase “Hit me up” means to contact or get in touch with someone, typically by phone or text message. The Chinese phrase “打我” means “hit me,” and it does not convey the meaning of the original phrase.
- Microsoft made bolder choices when it comes to the linguistic adaptation of the idioms.
- GPT had a harder time evaluating Microsoft’s metaphorical choices as they departed more.
- GPT-3 had an easier time with the Qualitative Analysis producing cogent textual analysis (even though with only 70% accuracy).
- Although intelligible GPT’s analysis failed to identify in 30% of the cases. This coincided with metaphorical choices that were literal and understandable but deviated from quotidian discourse.
- GPT-3 had a harder time translating the qualitative analysis into a score. Although broadly speaking scores were 60% accurate,it was difficult to differentiate between similar scores such as a 3 vs. a 4.
- Extreme score divergence from 1 to 5 was easier to understand and more compatible with overall comments suggesting that:
- Perhaps scoring criteria was not sufficiently calibrated with GPT-3
- Perhaps binary scoring could be more relevant than gradient scoring
- • Even though quantitatively Microsoft performed similarly to Google and Amazon, when you get into the nitty gritty of language, Microsoft made bolder choices and provided better results from a qualitative perspective but still was far behind GPT-3 when it came to accuracy and cultural adaptation. For example, The translation “我掌握了窍门。” is a clear and accurate translation of “I get the hang of it.” It conveys the meaning of the original phrase well and is easy for native Chinese speakers to understand. It is also a more figurative and idiomatic way of expressing the idea of understanding or mastering something compared to other possible translations.
Practical applications and limitations
In this analysis GPT-3 provided superior contextualization and adaptation than previous machine translation models.
While none of the engines are reliable enough to replace humans (at least in the context of this study), GPT-3 shows clear capability of aiding human translators and reviewers in the process of translating and evaluating language.
French: MT Engines Failed on 60% of Idioms—GPT Stood Out
The following analysis was written by our English/French linguist Laurène Bérard.

Overall all engines struggled with the metaphorical nature of language, often erring in excessive literality.
Output from a Qualitative Perspective
The table below presents a synthetic analysis of 10 English idiom sentences translated into French, as conducted by our team of linguists.
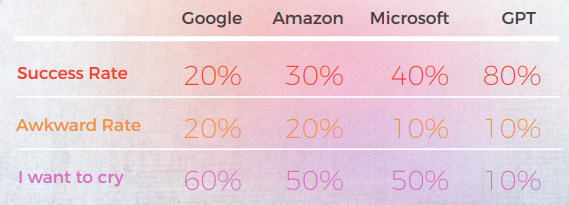
As far as translation quality goes, GPT did a great job with contextualization. Without any guidance, GPT was totally wrong for “The cat’s out of the bag” but was right with more guidance, and its 3 translations were grammatically incomplete thus quite difficult to understand for “It takes two to tango”.
The content was generally well adapted, intelligible, and conveyed the appropriate meaning. The three Machine Translation engines had a high “I want to cry” rate while even without context a translator would have guessed it wasn’t meant literally (e.g. “Let’s call it a day”, which is quite obvious but was totally misunderstood by Google engine).
The table below contains the raw data analysis.
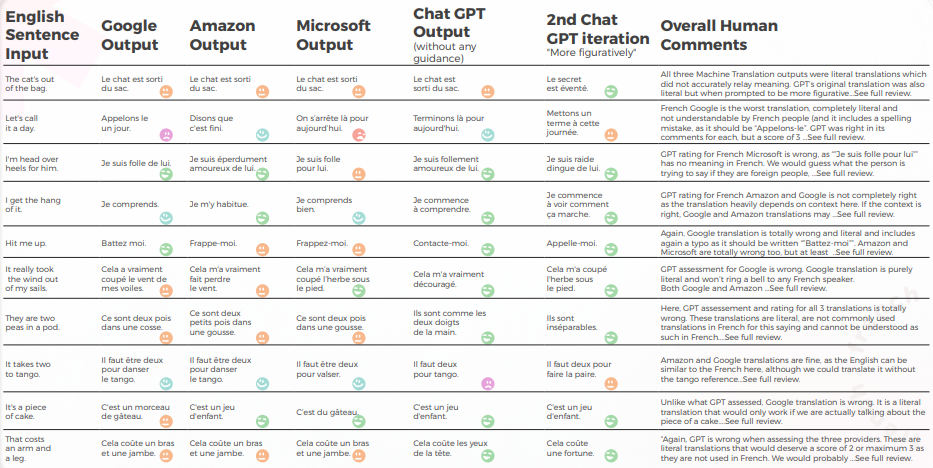
Evaluation of GPTs Evaluation
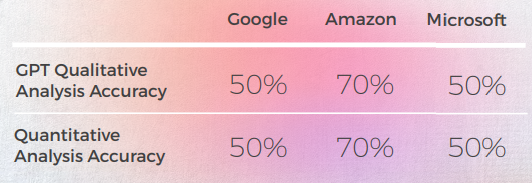
Analysis and Key Findings
- Despite translating correctly 8 times out of 10, GPT analysis of the 3 engines were often wrong, as almost 50% of the cases stated that their translation was fine while it was not.
- Although intelligible GPT’s analysis failed to identify issues in 30 to 50% of the cases, this coincided with choices that were literal and would have been correct if not dealing with idiomatic expressions.
- GPT failed to spot the two spelling issues from Google (“Appelons le” and “Battez moi”).
- GPT qualitative and quantitative analyses were globally consistent with each other.
- GPT had a harder time evaluating Microsoft’s and Google’s translations.
- Microsoft made better choices when it comes to the linguistic adaptation of the idioms.
- Google and Amazon had very similar results, only slightly deviating from each other. Microsoft stood out from the two.
- In one case (the tango expression), GPT evaluated a fine translation from Microsoft as being wrong (score of 2) while it was understandable and equally good as Google and Amazon translations which GPT evaluated positively (score of 5), but it may be explained by the fact that Microsoft was not as literal as the other two engines, as it chose to mention walz instead of tango.
- Microsoft made bolder choices and provided better results from a qualitative perspective but still was far behind GPT when it came to accuracy and cultural adaptation.
Practical applications and limitations
In this analysis GPT provided far better contextualization and adaptation than Amazon, Google and Microsoft machine translation engines. While it’s not convenient to replace traditional Machine Translation models with larger models such as GPT-3 due to high computational costs and diminishing marginal gains when it comes to non-metaphorical discourse, GPT-3 can be a powerful human ally when it comes to providing suggestions and identifying potential mistakes as well as opportunities for improvement.
Even though machine translation engines are “nearly” there, this “nearly” becomes progressively harder to tackle as proven by GPT here.
While none of the engines are reliable enough to replace humans (at least in the context of this study), GPT shows clear capability of aiding human translators and reviewers in the process of translating and evaluating language.
GPT Outperformed All MT Engines in German Idioms by 30%
The following analysis was written by our English/German linguist Olga Schneider.
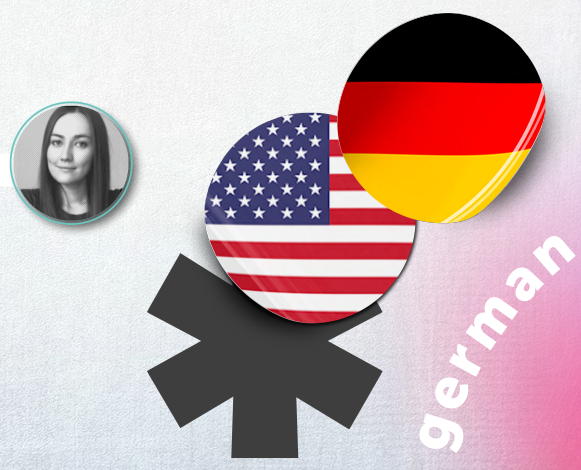
Overall, GPT produced the most accurate translation. It always analyzed the English sentence accurately and could usually tell whether a machine translation was literal or idiomatic, but it failed to detect a mistranslation 50% of the time.
Output from a Qualitative Perspective
The table below presents a synthetic analysis of 10 English idiom sentences translated into German, as conducted by our team of linguists.
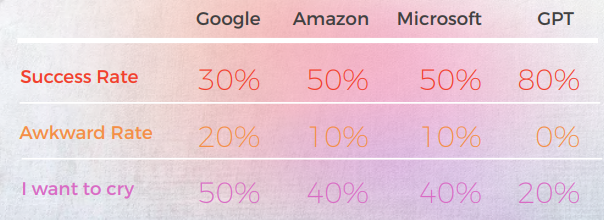
GPT produces good results 8 out of 10 times on the first try and 7 out of 10 times on the second try. For example, only GPT was able to provide accurate idiomatic translations for “I’m head over heels for him” and “Hit me up”.
The last, most figurative output produced 4 good sentences, with the others being either all right or “I want to cry”. Overall, Amazon and Microsoft produced slightly better translations than Google.
The table below contains the raw data analysis.
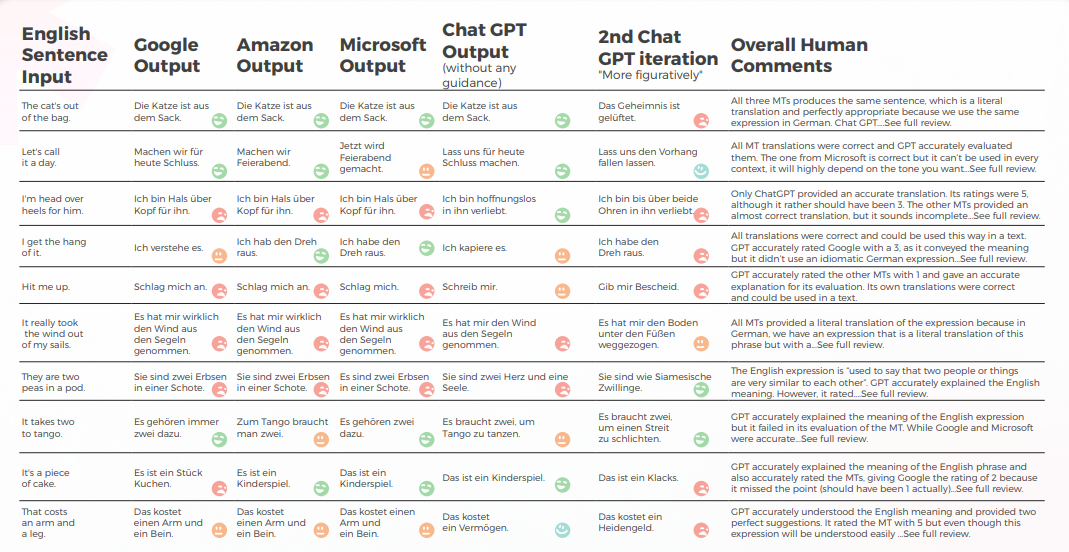
Evaluation of GPTs Evaluation
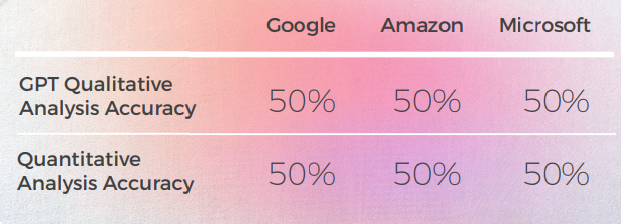
Analysis and Key Findings
- GPT has a fairly good knowledge of German idiomatic expressions, even the ones that did not quite match the English sentence were idiomatic expressions of a similar category. For example: “Let’s pull the plug” and “Let’s drop the curtain” for “Let’s call it a day”. It understands the general meaning of these phrases related to “ending” and could be a useful source of inspiration.
- When it comes to creative expressions, GPT seems to take inspiration from English phrases, suggesting the literal German translation of “I am in love with him from head to toe” as a creative alternative to “I’m head over heels for him”.
- GPTs translation ratings were hit or miss and proved to be unreliable. In one case, it rated a Google translation a 2, and it was not clear why it was not the 1 it should have been.
- German and English share many idiomatic expressions, which makes translation easier. But expressions that are foreign to German (e.g. “They are two peas in a pod” or “It’s a piece of cake”) end up being translated literally. In the case of the peas, unlike the translation engines, GPT understood that it needed to provide expressions about “twos”. However, the expressions it provided – while accurate and commonly used – didn’t convey the correct meaning.
Practical applications and limitations
GPT provided better translations than Google, Amazon and Microsoft. While it is not 100% reliable, it can provide a better starting point for machine translation editing than the other three engines. While idiomatic expressions are important, there is another problem often encountered in machine translation of English to German text: cumbersome sentence structure that is too close to the original text. It would be important to see how GPT solves this problem.
When it comes to evaluation, GPT is not a good tool, as its evaluation of German translations is only 50% accurate.
Three more things would have been interesting to see:
- Can GPT compete with DeepL for German? While GPT may provide good translations, DeepL produces good German translations and also offers a range of features that simplify the translation process (glossary terms translated correctly with the correct plural and case, one-click editing, autocomplete sentences after typing one or two words to speed up rephrasing). GPT’s translation needs to be significantly better than that of DeepL to make up for the lack of features.
- Could GPT’s accuracy be improved with more context, such as a paragraph containing the phrase?
- If Google is able to recognize AI-generated text, how will it handle GPT translations with minimal to no editing? Can it detect the “GPT style” and penalize a text in its search results?
In summary, in its current state, GPT can be a source of inspiration for our limited human brains. It can provide decent translations. More than that, it can help us rephrase overused expressions, find metaphors, and think outside the box.
Italian: MT Engines Returned Literal Translations in 70% of Cases
The following analysis was written by our English/Italian linguist Elvira Bianco.
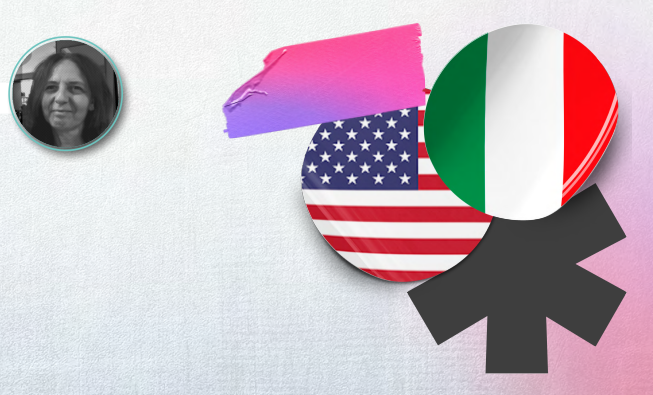
Overall all engines struggled with the metaphorical nature of language, often erring in excessive literality.
Output from a Qualitative Perspective
The table below presents a synthetic analysis of 10 English idiom sentences translated into Italian, as conducted by our team of linguists.
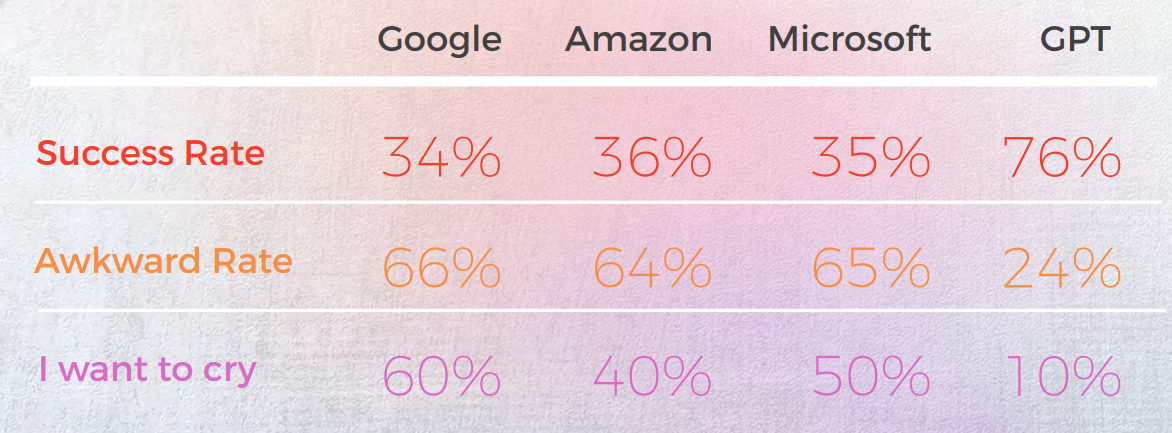
Machine translation gave a literal translation very far from the correct meaning. GPT conveyed the correct meaning and expression at 70%, for the remaining 30% used acceptable expressions that are not widely used or perceived like natural native speech.
The table below contains the raw data analysis.
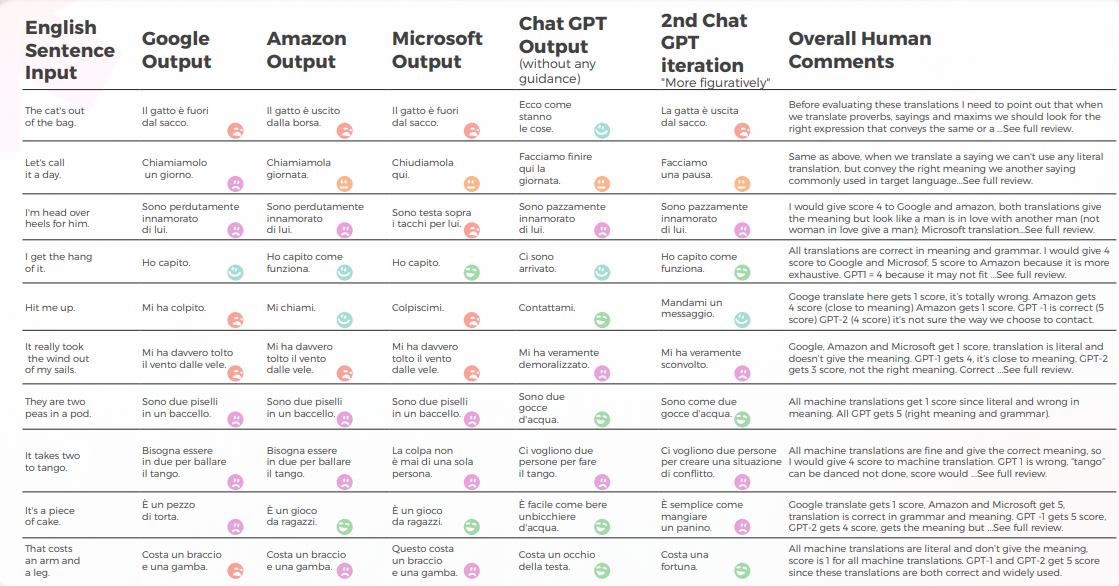
Evaluation of GPTs Evaluation
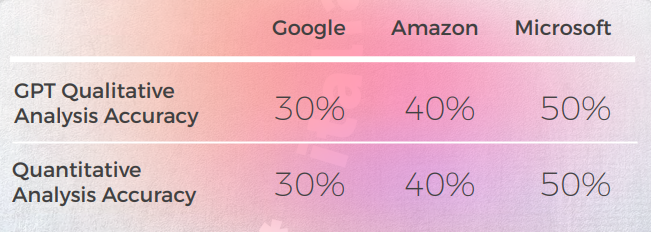
Analysis and Key Findings
- Google Translate gets only one right translation and gets close to meaning in 2 sentences.
- Amazon gave 50% right meaning even if not using the most common way to convey the English saying in Italian language.
- Microsoft gave 3 right answers getting closer to Italian similar sayings.
- While 1st Chat GPT usually approves machine translations, 2nd and 3rd Chat GPT usually give the correct meaning and add valuable translation suggestions.
Practical applications and limitations
As defined by https://it.wiktionary. org/wiki/espressione_idiomatica an idiomatic expression typical of a language is usually untranslatable literally into other languages except by resorting to idiomatic expressions of the language into which it is translated with meanings similar to the idiomatic expressions of the language from which it is translated. Clearly the mechanical translation produced by today’s most used translation machines (Google, Amazon, Microsoft) was unreliable, nothingstanding GPT-3 proved to be able at 70% to give the right meaning and to furnish good suggestions in content adaptation.
It is not unlikely that in the near future machines will also memorize idiomatic expressions but right now we need humans to translate conveying the same meaning from one language to another.
Languages are full of nuances, double entendres, allusions, idioms, metaphors that only that only a human can perceive.
Korean Had the Lowest Accuracy Across All Engines Tested
The following analysis was written by our English/Korean linguist Sun Min Kim.
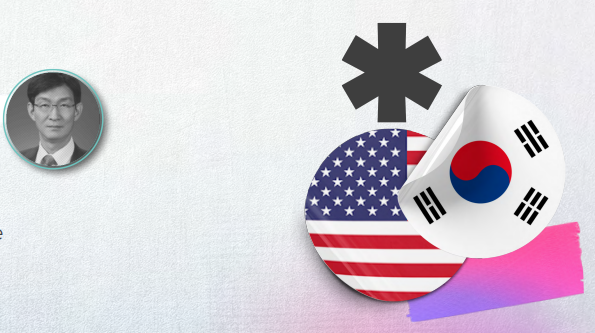
Output from a Qualitative Perspective
The table below presents a synthetic analysis of 10 English idiom sentences translated into Korean, as conducted by our team of linguists.
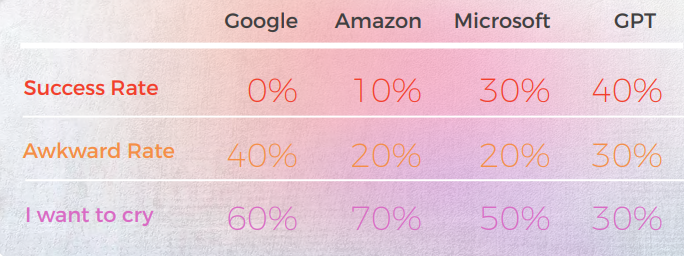
Most engines literally translate the idiomatic expressions, while GPT tries to translate as descriptive as possible using no metaphor (e.g. piece of cake = easy, while in Korea, we have a similar idiomatic expression that conveys the same meaning as Microsoft did.
GPT’s three translations are not consistent. Some are getting worse with the iteration.
The table below contains the raw data analysis.
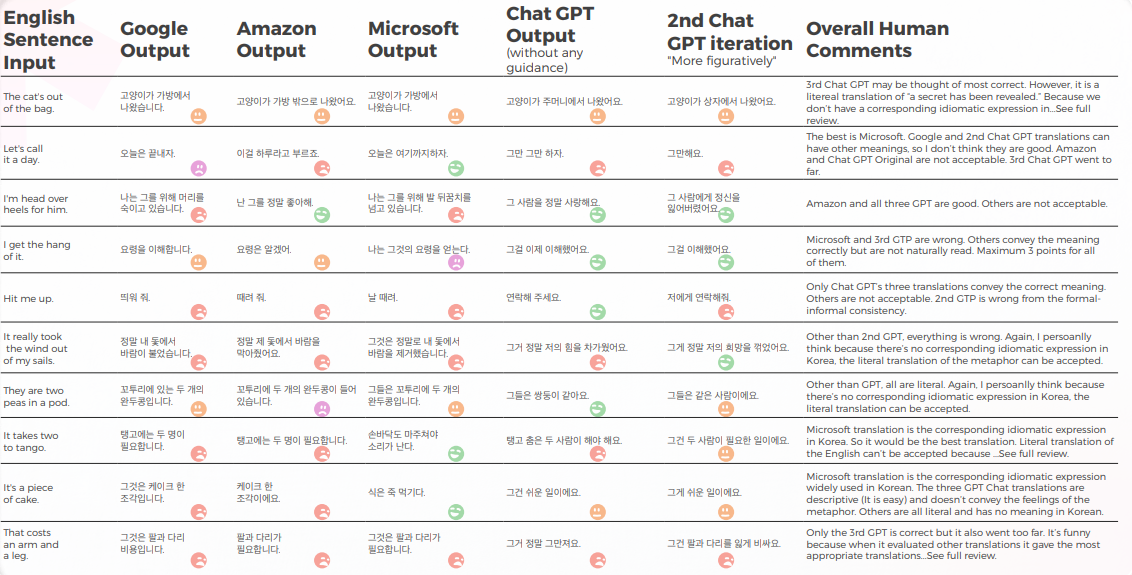
GPT knows the problems when the translations go wrong. But it is not considered to be correct in judging the best one.
GPT itself has issues with the formal – informal treatment and so on) and it can evaluate this issue.
Evaluation of GPTs Evaluation
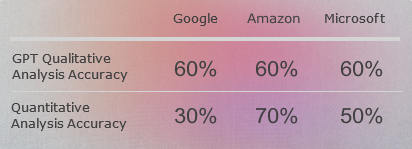
Analysis and Key Findings
Because the English originals in this study are idiomatic expressions, it is a little bit tricky because you have to choose between the metaphor or direct description. But for some idiomatic expressions where the Korean language has similar idiomatic expression conveying the same meaning, most of the engines missed to find those expressions with only few exceptions (please see the worksheet and find those with the score of 5 by me).
For others, my personal thought is that if the metaphor itself can convey the meaning, literal translation of it can be considered and maybe better to use a descriptive word. Of course, if the metaphor has no cultural context in Korea, it should not be literally translated. But it’s a subtle issue and maybe up to the preference or human emotions of the translator. I don’t think any engine has that level of human-like thinking yet.
Practical applications and limitations
I do think most of the engines can be used for pre-translation purposes. But considering the quality, it should be primarily for the efficiency purpose only (that is, not typing from the scratch). For more descriptive texts, such as manuals, I see MTPE is much more advanced than these idiomatic expressions. So, there is still room to improve.
GPT Hit 90% Success Rate in Brazilian Portuguese Idioms
The following analysis was written by our English/ Portuguese linguist Gabriel Fairman.
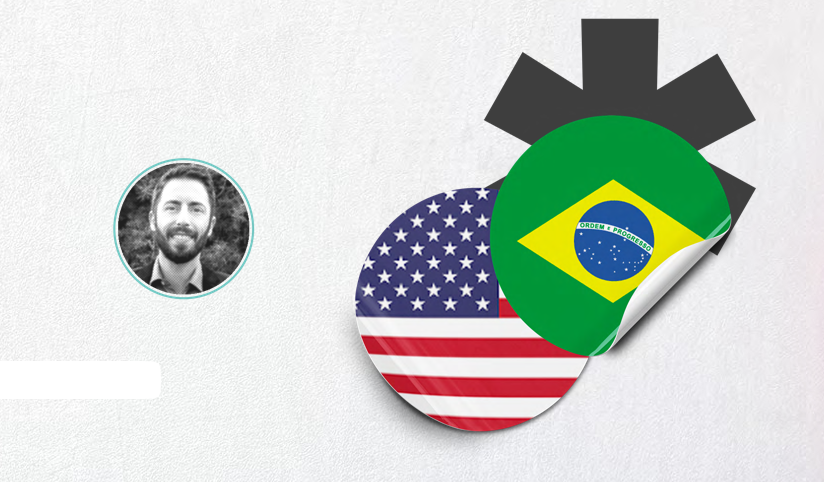
Overall all engines struggled with the metaphorical nature of language, often erring in excessive literality.
Output from a Qualitative Perspective
The table below presents a synthetic analysis of 10 English idiom sentences translated into Portuguese, as conducted by our team of linguists.

Most engines literally translate the idiomatic expressions, while GPT tries to translate as descriptive as possible using no metaphor (e.g. piece of cake = easy, while in Korea, we have a similar idiomatic expression that conveys the same meaning as Microsoft did.
GPT’s three translations are not consistent. Some are getting worse with the iteration.
The table below contains the raw data analysis.
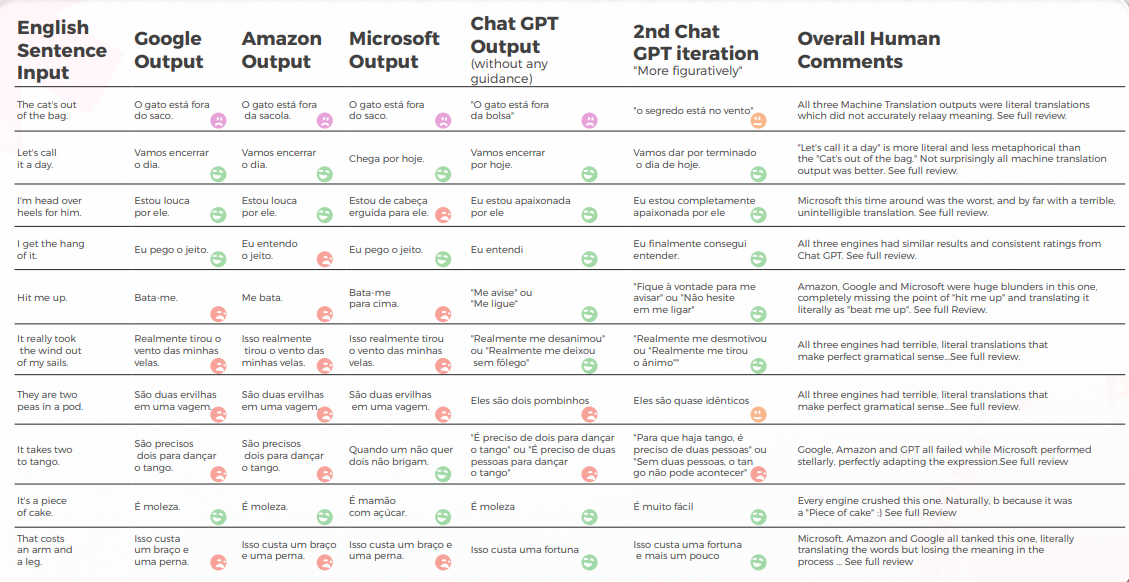
As far as translation quality goes, GPT did a great job with contextualization. In 9 out of 10 sentences, the content was well adapted, intelligible, and conveyed the appropriate meaning. Contrary to the three Machine Translation engines, GPT had no embarrassing “I want to cry” mistakes.
The initial hypothesis was that there would be a big difference in quality between GPT’s first and second iterations, but translation quality was similar in both.
Evaluation of GPTs Evaluation
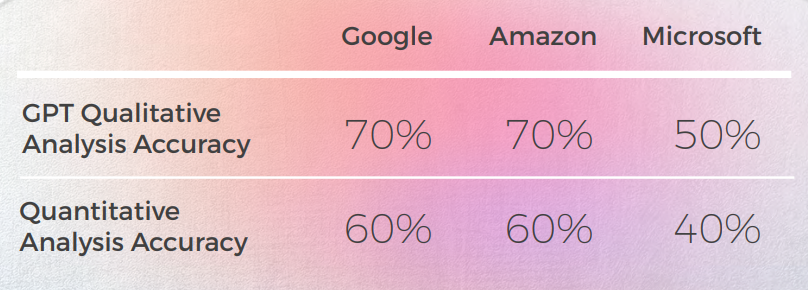
Analysis and Key Findings
- Microsoft made bolder choices when it comes to the linguistic adaptation of the idioms.
- GPT had a harder time evaluating Microsoft’s metaphorical choices as they departed more.
- Google and Amazon had extremely similar results, only slightly deviating from each other, mirroring each other’s mistakes and metaphorical choices. Microsoft clearly stood out from the two.
- GPT-3 had an easier time with the Qualitative Analysis producing cogent textual analysis (even though with only 70% accuracy).
- Although intelligible GPT’s analysis failed to identify in 30% of the cases. This coincided with metaphorical choices that were literal and understandable but deviated from quotidian discourse.
- GPT-3 had a harder time translating the qualitative analysis into a score.
- Although broadly speaking scores were 60% accurate,it was difficult to differentiate between similar scores such as a 3 vs. a 4.
- Extreme score divergence from 1 to 5 was easier to understand and more compatible with overall comments suggesting that:
- Perhaps scoring criteria was not sufficiently calibrated with GPT-3
- Perhaps binary scoring could be more relevant than gradient scoring In one anomalous case Cchat GPT- 3 evaluated two similar translations in radically different ways giving one a 1 and the other a 5 when both of them should have been 1.
- Even though quantitatively Microsoft performed similarly to Google and Amazon, when you get into the nitty gritty of language, Microsoft made bolder choices and provided better results from a qualitative perspective but still was far behind GPT-3 when it came to accuracy and cultural adaptation.
Practical applications and limitations
In this analysis GPT-3 provided superior contextualization and adaptation than previous
machine translation models in Brazilian Portuguese. While it’s not convenient to replace traditional Machine Translation models with larger models such as GPT-3 due to high computational costs and diminishing diminishing marginal gains when it comes to non-metaphorical discourse, GPT-3 can be a powerful human ally when it comes to providing suggestions and identifying potential mistakes as well as opportunities for improvement.
Linguistic edge cases are amazing because they illustrate so clearly how much is left in so little when it comes to language models. Even though they are “nearly” there, this “nearly” becomes progressively harder to tackle and if not harder, definitely more expensive from a computational perspective.
While none of the engines are reliable enough to replace humans (at least in the conext of this study), GPT-3 shows clear capability of aiding human translators and reviewers in the process of translating and evaluating language.
Spanish MTs Scored Under 30%—GPT Was the Clear Winner
The following analysis was written by our English/ Spanish linguist Nicolas Davila.
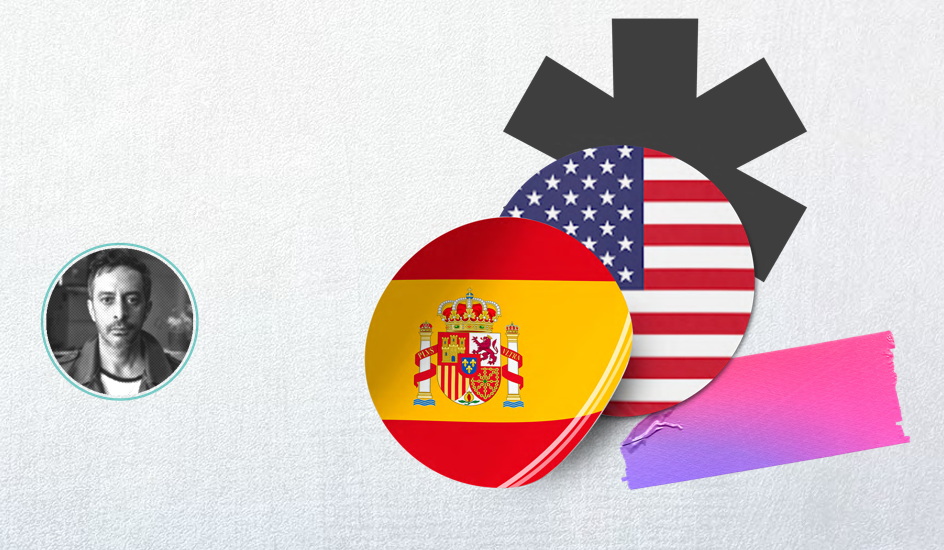
Overall all engines struggled with the metaphorical nature of language, often erring in excessive literality.
Output from a Qualitative Perspective
The table below presents a synthetic analysis of 10 English idiom sentences translated into Spanish, as conducted by our team of linguists.

As far as translation quality refers, GPT did an acceptable job and better than the others with contextualization. In 7 out of 10 sentences, the content was intelligible, well formed, and conveyed the appropriate meaning, with low awkwardness and “I want to cry” rates.
Although the initial hypothesis was that there would be a big difference in quality between GPT’s first and subsequent iterations, translation quality is similar in all of them, 2nd and 3rd iterations sometimes add unnecessary stuff, rising a bit the awkward rate.
The table below contains the raw data analysis.
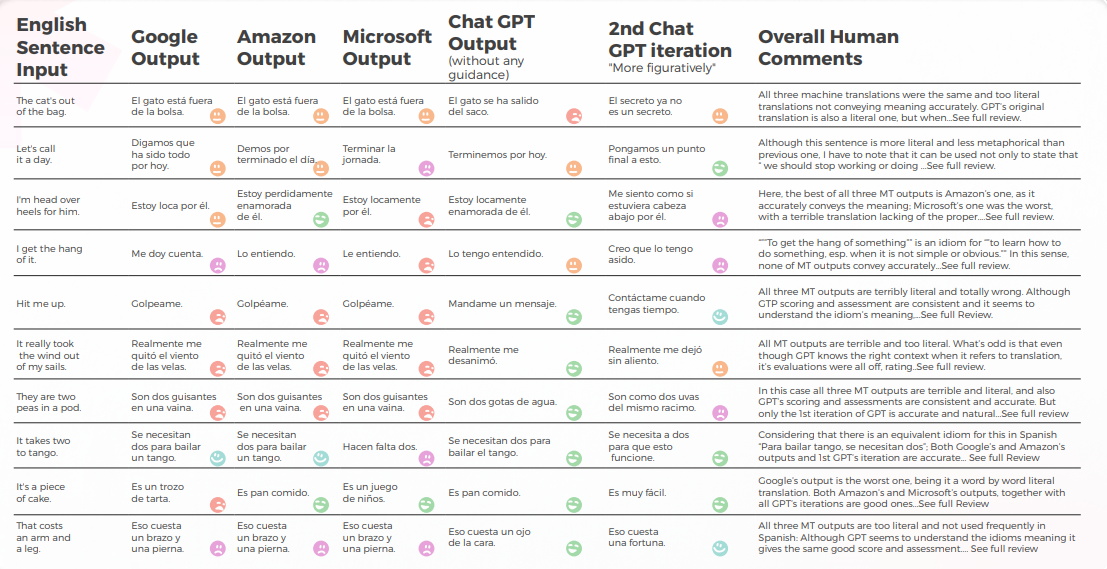
Evaluation of GPTs Evaluation
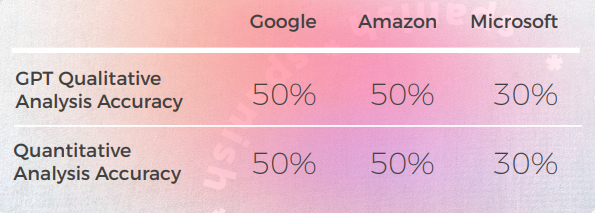
Analysis and Key Findings
- MT translations were too literal, being Amazon and Google very similar in general terms, and Microsoft being the worst one.
- Google and Amazon had extremely similar results, only slightly deviating from each other, mirroring each other’s mistakes and metaphorical choices. Microsoft performed poorly, sometimes producing sentences ill formed and missing some parts of the grammatical construction.
- GPT-3 had an easier time with the Qualitative Analysis producing coherent textual analysis, even though with only 50% accuracy.
- Frequently, GPT-3 qualitative analysis was too general and more restricted to the main and literal meaning of the sentence, without considering subtle details of construction and change in meaning. It seems GPT is not able to catch such differences and to translate them into quantitative scores.
- Also, GPT frequently assigned the same qualitative analysis and high quantitative score to sentences that were grammatically poorly constructed, which seems to be a limitation of GPT’s model.
- GPT-3 had a harder time translating the qualitative analysis into a score.
- Being it only 50% accurate for Google and Amazon and only 30% accurate for Microsoft. It seems GPT was only measuring if the sentence conveyed the meaning, but no differences in construction or well formation.
- Score divergence from 1 to 5 was easier to understand and more compatible with overall comments suggesting that: Perhaps scoring criteria was not sufficiently calibrated for GPT-3 Perhaps binary scoring could be more relevant than gradient scoring. Perhaps scoring criteria or GPT model were not considering grammatical issues, but only conveying meaning.
Practical applications and limitations
In this analysis GPT-3 provided superior contextualization and adaptation than previous machine translation models in Latam Spanish.
GPT-3 could be a powerful tool in helping humans to improve translations when it refers to providing useful suggestions and opportunities for improvement. But, as far as I can see, it still has certain limitations.
Although larger models such as GPT-3 could be helpful, it is not convenient to replace traditional Machine Translation Models with them, due to higher computational costs, as when it comes to non- metaphorical text it could diminish marginal gains.
While GPT-3 shows clear capability of aiding human translators and reviewers in the process of translating and evaluating language, cost considerations should be included when evaluating its use for non-metaphorical texts.
Conclusions
While ChatGPT’s performance can vary depending on the prompt and the specific language, our study suggests that ChatGPT has the potential to produce higher quality translations than traditional MT engines, especially when it comes to handling idiomatic expressions and nuanced language use.
However, it is important to note that ChatGPT is far from not making mistakes and still has immense room for improvement, especially when it comes to more complex prompts or language domains.
As a translator, ChatGPT was more successful than all tested Machine Translation engines. While languages showed different results, Korean was clearly the outlier with MT quality and GPT quality significantly lower than other languages.
In all languages except Korean, ChatGPT had at least a 70% success rate and at most a 90% success rate, performing better than traditional MT. And even in Korean, while scores were low, they still were better than MT engine output.
Contrary to Machine Translation, with an LLM, Iterations of the same content can improve the output quality. This is key when thinking about integrations because whereas with traditional MT your output will always be the same to your input (unless the engine gets further data or training), with an LLM one can explore several interactions via API in order to optimize feed quality.
One advantage of ChatGPT over traditional MT engines is its ability to learn and improve over time, even without additional training data. This is due to the nature of LLMs, which are designed to continually refine their language models based on new input. As such, ChatGPT can potentially offer more adaptive and dynamic translation capabilities, which could be especially useful in scenarios where the language or content is constantly evolving or changing.
Another advantage of ChatGPT is its low cringe rate, which is a significant improvement over traditional MT engines that often produce awkward or inappropriate translations. This could make ChatGPT more acceptable and user-friendly for non-expert users who may not have the same level of linguistic or cultural knowledge as professional translators.
However, it is important to note that ChatGPT is not a substitute for human translators, and there are a myriad of cases where the expertise and judgment of a human translator are needed. But ChatGPT’s significantly lower cringe rates opens the door for a wider adoption of non-human driven translations.
As an evaluator, ChatGPT’s performance was more mixed, with accuracy rates ranging from 30% to 70%. While this suggests that ChatGPT may not be as effective at evaluating other translation engines as it is at suggesting translations, it is possible that this is due to the complexity and quality of the evaluation prompts, which may require more specialized or contextual knowledge than ChatGPT currently possesses. Further research is needed to explore ChatGPT’s potential as an evaluator, as well as its limitations and challenges.
Overall, our study suggests that ChatGPT has promising translation capabilities that are worth exploring further. While it may not be able to replace or bypass human translators entirely, it could potentially offer significant benefits as an aid or pre-translation tool, especially in scenarios where time, resources, or expertise are limited.
As with any emerging technology, there are still many challenges and opportunities for improvement, and further research and experimentation will be needed to fully unlock its potential.
Methodology
To explore how language models like GPT-3 handle idiomatic translation compared to traditional machine translation engines, we conducted an experiment focused on linguistic edge cases involving metaphor and idiomatic expressions.
Selection of Idiomatic Expressions
We selected 10 commonly used English idioms, such as “Let’s call it a day,” “Hit me up,” and “The cat’s out of the bag.” These expressions are well known for challenging translation systems, as they require cultural and contextual adaptation rather than literal equivalence.
Translation Engines and Models Tested
Each idiom was translated using:
- Google Translate
- Amazon Translate
- Microsoft Translator
- GPT-3 (ChatGPT) – using two approaches:
- A direct translation without any guidance
- A second version prompted to be more figurative and idiomatic

Evaluation Process
The translated outputs were reviewed by native-speaking linguists across seven target languages: Brazilian Portuguese, Spanish, French, German, Italian, Chinese, and Korean. For each sentence, reviewers evaluated:
- Whether the meaning of the original idiom was preserved
- The naturalness of the expression in the target language
- Grammar, syntax, and semantic accuracy
Each translation was rated on a 1 to 5 scale:
- 5: Correct meaning and natural-sounding language
- 1: Incorrect, confusing, or unnatural translation ("I want to cry" level)
Additionally, we asked ChatGPT to evaluate the other engines’ translations, both by providing qualitative textual feedback and numerical scores. This allowed us to assess its capability as an evaluator, not just as a translation generator.
Study Limitations
It’s important to note that this was an exploratory study:
- The sample was limited to 10 idioms with one human reviewer per language.
- All evaluations carry a degree of subjectivity and individual preference.
- GPT-3’s performance reflects a specific version of the model and may evolve with updates.
Despite these constraints, the findings provide valuable insight into the capabilities and limitations of current models, especially in linguistically nuanced and culturally dependent scenarios.
Disclaimers
(Real research disclaimers not to be taken lightly)
While we aimed to explore the potential of ChatGPT’s language capabilities, it is important to note that this study only evaluated one aspect of translation, namely the ability to handle linguistic idiomatic highly metaphorical edge cases. Other aspects of translation, such as cultural and contextual understanding, may require different evaluation methods and criteria.
The sample size of this study is limited to 10 idioms and one reviewer per language, which may not be representative of the full range of idiomatic expressions in the English language, or the range of perspectives and expertise of professional translators. As such, the results of this study should be interpreted with caution and cannot be generalized to other contexts or domains.
Furthermore, the opinions and evaluations of the single reviewer per language are subjective and may be influenced by personal biases, experiences, or preferences. As with any subjective evaluation, there is a degree of variability and uncertainty in the results. To increase the reliability and validity of our findings, future studies could involve multiple reviewers, blind evaluations, or inter-rater reliability measures.
It is also worth noting that ChatGPT’s language capabilities are not static and may change over time as the model is further trained and fine-tuned. Therefore, the results of this study should be considered as a snapshot of the model’s performance at a specific point in time, and may not reflect its current or future capabilities.
Lastly, this study is not intended to make any definitive or categorical claims about the usefulness or limitations of ChatGPT for translation. Rather, it is meant to serve as a preliminary investigation and starting point for future research and development in the field of natural language processing and machine translation. As with any emerging technology, there are still many challenges and opportunities for improvement, and further experimentation and collaboration will be needed to fully explore its potential.