Context-aware translation with Large Language Models
Traditional machine translation has been problematic for a long time, even with the introduction of neural machine translation and large training data sets. Although it can produce interesting results when finely tuned and trained for a specific domain with predictable and straightforward language, it is generally unreliable and erratic when applied to a variety of domains, languages, and circumstances.
Many reputable translators still dislike machine translation and refuse to use it as a first draft or rewrite the feeds entirely. This highlights the divide between machines and humans, even with the immense evolution of machine translation in recent years. Based on our polling, only a small percentage of translators consider machine translation to be a valuable ally.
Traditional machine translation is either too generic and erratic or too specific, requiring specific circumstances to be effective, such as large volumes of content with simple linguistic structure or domain segregation like technical manuals, product knowledge-base, and support literature. Even trained engines struggle with handling inconsistencies and discrepancies between term bases, translation memories, and linguistic corpus training.
These include updated glossaries by company reviewers or translators after the training process, differences between glossaries and training corpus, the need to create and maintain specific engines for maximum quality, translation memory deviations from the trained corpus, and silly mistakes such as translating entities or other proper nouns, and lack of cultural or linguistic sensitivity.
These examples result in unreliable feeds and a challenging review process for translators. Additionally, managing tuned and trained machine translation models typically requires one or more localization engineers dedicated to these efforts, something that most small and medium-sized translation agencies and localization programs do not have the bandwidth for.
Context-aware translation with Large Language Models, however, changes this. Context refers to all information that is not the text itself but helps the engine make sense of the text and how to process it. Examples of context are glossaries, translation memories, past feedback, and bounce rate, but context could be any information. With Large Language Models that can take into account billions of parameters by design, there really is no limit to how much context you decide to work with.
Here is an example of taking context into account:

Note how there is a 89% Translation Memory Feed and a Machine Translation Feed. The TM feed calls our “engine” a “motor” in Portuguese while the MT calls the engine a “mechanism” in Portuguese. The glossary however specifices that “engine” should be kept as “engine”. When processed through Bureau Works Translate, our model takes this preference (along with others) into account:
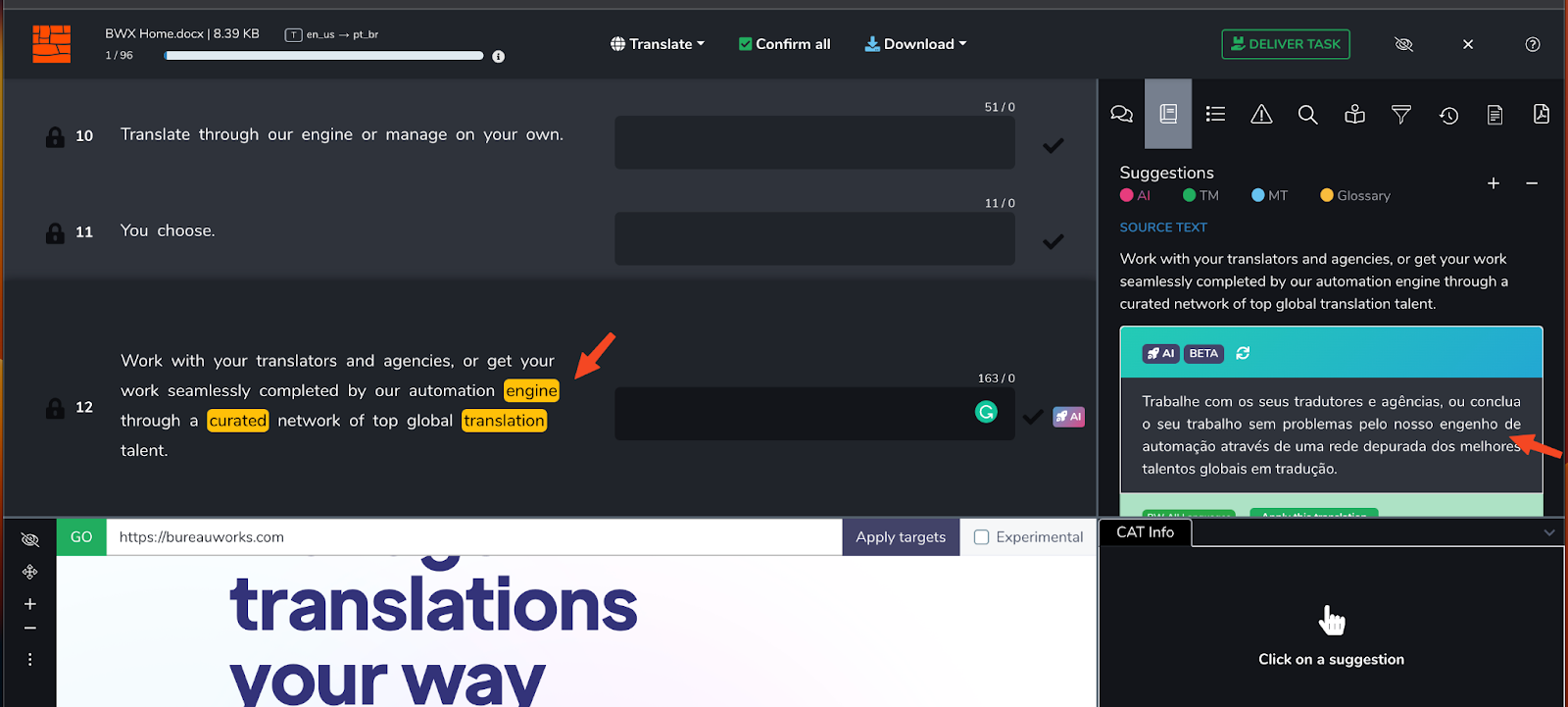
Bureau Works Translate not only inserts the correct term according to the latest glossary version but makes any necessary changes to the text so that it reads well in the target language while also factoring in linguistic context offered by the Translation Memory.
With Bureau Works Translate, Large Language Models like ChatGPT can take into account their own training data set, specific translation memories, glossaries, and other relevant context to offer translators a nuanced, contextualized, and mindful translation feed in a given project. We can take into account the latest updates to glossaries and translation memories in real-time, which means there is no need to submit updated glossaries and translations repeatedly to trained and tuned machine translation servers to hope they factor in the linguistic preferences.

With Bureau Works Translate, you can train and translate on the fly, and any translation project, any-sized translation memory, and any-sized glossary can benefit from its textual merging and linguistic probabilistic capabilities. There is no setup time and no need to train beforehand.
Based on our initial research, such an engine can provide translators with feeds that break the long-standing resistance towards leveraging machine output. Our engine also opens the door for a conversation between the translator and the engine. It makes more sense to enrich a glossary if you benefit from it instantly, and it is easier to trust a machine if you know from experience that there is at least something that resembles critical and adaptive thinking.
In addition to the Context-aware translation, we have also opened the door for translators to interact with language models so that they can get a second pair of eyes on their choices, alternative suggestions, and even start a conversation when needed.
Context-aware translation with Large Language Models is more than an improved pre-translation process. It's a firm step towards humans working with machines to produce better content with less effort and in less time.
Moving forward the context will continue to expand in unprecedented ways and we will be able to author multilingual texts taking into account user behavior, web analytics, and a plethora of other information bodies that can be consumed and understood by large language models. This is only the very beginning and it’s already changed everything.